The Artificial Intelligence Channel
Dr. Blake Richards is an Assistant Professor at the University of Toronto and Associate Fellow of the Canadian Institute for Advanced Research (CIFAR). Recorded May 2nd, 2018 at ICLR2018
Source
Deep Learning with Ensembles of Neocortical Microcircuits – Dr. Blake Richards
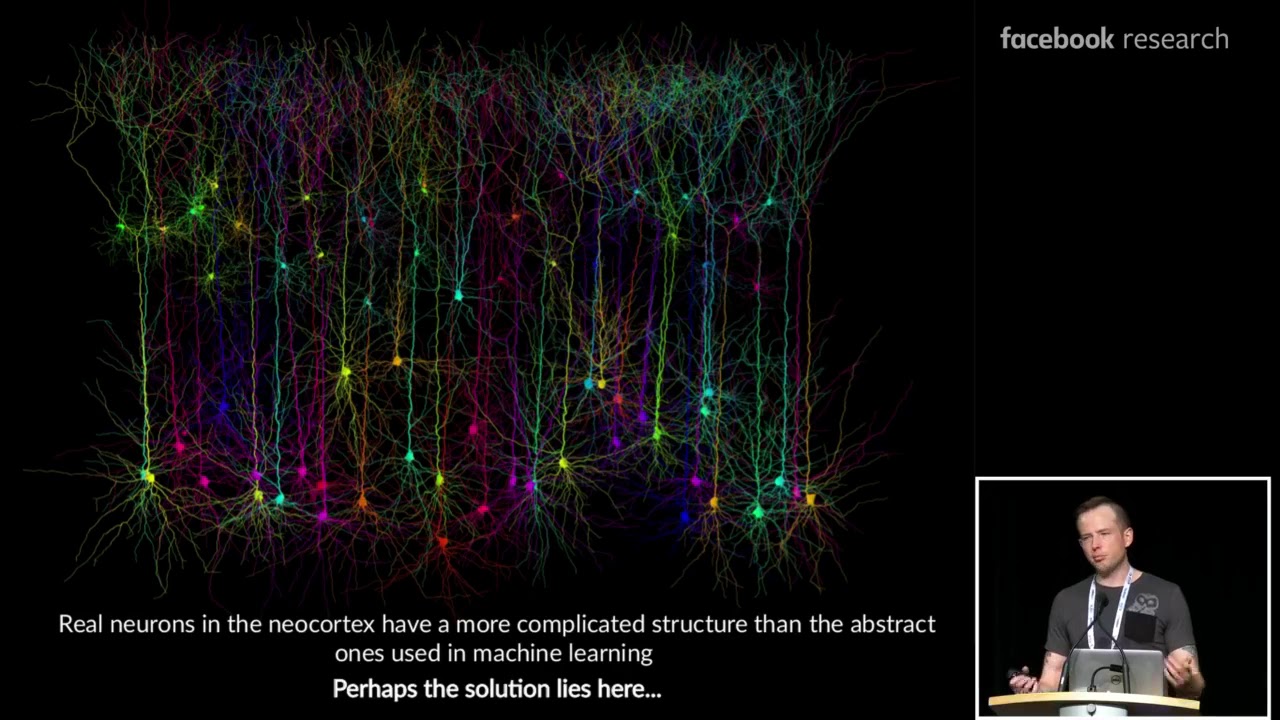
The mitochondria is the power house of the cell.
Fascinating.
I wonder if this work will give any insight into excitotoxicity. Understanding sodium / potassium channels and especially the role of magnesium inside the neuron would be super useful in understanding toxic withdrawal of GABA agonists like benzodiazepines and alcohol.
Link to the paper?
Wow, this is a fundamental change on how to build a neural network, for such kind of new neural network, we will call it "Neurons Group Neural Network", NGNN for short š
I would strongly suggest that the difference between learning and thinking is as profound and great as the difference between brain and mind. In both cases, studying the former provides no insight into the latter. From the very beginning of " studies into AI", mind has been erroneously conflated with brain. The longer this error persists within the industry, the greater the risks to the human species.
Your eyes are not "learning" how to see. This is the problem with trying to equate the function of deep neural networks in computers to human organs which have evolved over millions of years. The visual cortex is designed to capture the features of the real world in high fidelity. Meaning it is designed to capture the light, shadow, texture, shapes and other forms of 'ground truth' and reassemble that into a bio-organic chemical signal that gets passed on to other parts of the brain. "Feature extraction" and "inference" in a biological context is not the same as what we see in modern Neural networks. Therefore, your visual cortex is extracting features such as "light and shadow", "surface texture", "orientation", "perspective" and "dimensional data" from a visual perspective as "features" of the real world. The real world has light and shadow, these are features along with color and texture and perspective/dimensions. And all of those "features" are passed along together through multiple neural pipelines, almost equivalent to hundreds or millions of mini-gpu pipelines that are passing texture info, color info, lighting info and other info along multiple parallel chains of neurons up to the higher organs of the brain. The key difference here is that this data is not converted simply into a statistical result. The fidelity is maintained and stored together at all times. Hence the better your memory, the more "photographic' it is as in the more details you have been able to recall from past events.
The main benefit of all these massive pipelines of visual processing is that there are multiple "features" of the visual world that can be extracted and processed an abstracted by the higher order organs of the human brain where "learning" occurs. And because of the fidelity and complexity in the features passed through the circuits of the visual cortex, you learn to recognized objects in a relatively short time, because these circuits operate in real time, capturing thousands of frames of visual data per second. Computer neural nets in no way can replicate this because computer neural networks are to resource hungry and based on statistical models that throw away all the important visual details and fidelity in order to produce a statistical result.
So when you talk about human image recognition the reason it works so quick and without requiring thousands and thousands of passes is because of the biological and physical characteristics of how human eyes capture and convert visual light into chemical signals. Rods and Cones in the eyes do different things. Therefore each neural pathway is inherently dealing with different "features" associated with visual data. It is not all together in a "single bucket" as in a single 2d image which you see on computer. Hence much of the feature extraction has already taken place just as a function of the optical nerves in transcoding visual data. And all this data is processed in parallel, which means you recognize things based on their texture, color, shape and other characteristics all together at the same time in real time because that is how you experience the real world through your eyes (in real time) and the feature extraction and breakdown is happening at real time. (Inferencing in humans is more like looking at how the light and shadow falls across a surface in "inferring" what the material is. IE, fur looks different from concrete and so forth.) You cannot currently do any of this in neural networks (single pass high fidelity multiple component visual feature extraction and detection) and certainly there is no computer system that can store the amount of data captured by the human eye in a single minute let alone a whole hour or lifetime.
I spent hours across months and approx 40gb data" conducting my own personal "deep learning". My devices were attacked by gamers, thwarting my incredible AI experience. "Let the magic begin".
Pretty pictures
I didn't understand any of it, but I love it.
interesting work, but where do I find some c/c++ source code?
He lost me and lām a neuroscientist.
Wow. "Backpropagation is not realistic in the context of the real biological brain".
Well, that's because the brain does it better – as this vid describes.
A blockbuster. Hinton is smiling.
Fascinating. And thank you for such a lucid presentation.
The guy who introduced him, anybody knows what's his name?
Anyone here also from the HTM community?
So like capsuke networks ?
So my takeaways are that
(1) Backprop does actually approximate complex neuronal behavior
(2) Neurons are organized and behave like a Capsule Network
(3) Third-party inhibitors are a motif worth investigating
I think (1) follows from their use of averaged hidden layer activity and simple loss differences. I can imagine that this converges to standard backprop, because averaging / differencing doesn't usually change behaviors in the limit.
The evidence for capsule network architecture didn't seem to flow from the previous topic. Yes simultaneous forward/backward passing because of the voltage threshold stuff, but why capsules? Did I miss it?
As for (3), this seems cool, especially with respect to capsule networks. However I remember dropout and weight decay being framed as inhibition already. I wouldn't be surprised to see inhibitor capsules converge to weight decay in the limit.
32:32
This give some insight into a greatly simplified hardware implementation of learning networks: no back-prop, linear activation, etc.
At 46:50 neurons involved when rewards occur, emotional centers?
This is amazing. Not the same old yadda yadda … nice to see some novel thinking in this space. multidisciplinary skills help too š
this kind of research can really push the boundaries
This talk made me dumber….I know now more things i know nothing about. ??
A video game with characters that are real ai characters making their own choices talking saying whatever they want to say.
Are the results of 31:10 published in a paper?
Great lecture. And then you realize…That the most sad thing on all this AI neural network business is that… all this could have been done DECADES AGO (many models devised, architectures designed, theoretically studied and partially implemented even on the low power hardware of the particular era)… but we had these "AI winters" (as many pioneers point out, at various points in time you were viewed almost as an astrology-level charlatan when STILL doing these idiotic neural networks… yep, good old inquisition as everyone expects in science). And we still do not know how many breakthroughs (or at least important new ideas) wee need. Some say that our beloved NewEvil internet monopolists (GOOLAGs of this world) are actually actively creating new AI winter by sucking all the brains into their stupid cat-finding applications that use "whatever works" to generate tonZ of ad moneyZ (yep, no tech giants, they sell NO technology whatsoever, just ads and souls of their sheeple users).