Tejas Coursera
The rise of AI has
been largely driven by one tool in AI called
machine learning. In this video, you’ll learn what is machine learning,
so that by the end, you hope we will start
thinking how machine learning might be applied to your company
or to your industry. The most commonly used type of machine learning
is a type of AI that learns A to B, or input to output mappings. This is called
supervised learning. Let’s see some examples. If the input A is an email and the output B one is
email spam or not, zero one. Then this is the core piece of AI used to build a spam filter. Or if the input is an audio clip, and the AI’s job is to
output the text transcript, then this is speech recognition. More examples, if you want to input English and have it
output a different language, Chinese, Spanish, something else, then this is machine translation. Or the most lucrative form
of supervised learning, of this type of machine learning maybe be online advertising, where all the large
online ad platforms have a piece of AI that inputs
some information about an ad, and some information about you, and tries to figure out, will you click on this ad or not? By showing you the ads you’re
most likely to click on, this turns out to
be very lucrative. Maybe not the most
inspiring application, but certainly having
a huge economic impact today. Or if you want to build
a self-driving car, one of the key pieces of AI is in the AI that takes
as input an image, and some information from their radar, or
from other sensors, and output the position
of other cars, so your self-driving car
can avoid the other cars. Or in manufacturing. I’ve actually done
a lot of work in manufacturing where you take as input a picture of something
you’ve just manufactured, such as a picture of a cell phone coming
off the assembly line. This is a picture of a phone, not a picture taken by a phone, and you want to output, is there a scratch,
or is there a dent, or some other defects on this thing you’ve
just manufactured? And this is visual inspection
which is helping manufacturers to reduce or prevent defects in the things
that they’re making. This set of AI called
supervised learning, just learns input to output, or A to B mappings. On one hand, input to output, A to B it seems quite limiting. But when you find
a right application scenario, this can be incredibly valuable. Now, the idea of
supervised learning has been around for many decades. But it’s really taken off in the last few years. Why is this? Well, my friends asked
me, “Hey Andrew, why is supervised
learning taking off now?” There’s a picture
I draw for them. I want to show you
this picture now, and you may be able to
draw this picture for others that ask you
the same question as well. Let’s say on
the horizontal axis you plot the amount of data
you have for a task. So, for speech recognition, this might be the amount of audio data and
transcripts you have. In lot of industries, the amount of data
you have access to has really grown over
the last couple of decades. Thanks to the rise
of the Internet, the rise of computers. A lot of what used to
be say pieces of paper, are now instead recorded
on a digital computer. So, we’ve just been getting
more and more data. Now, let’s say on
the vertical axis you plot the performance of an AI system. It turns out that if you use
a traditional AI system, then the performance
would grow like this, that as you feed in more data is performance gets a bit better. But beyond a certain point it did not get that much better. So it’s as if your
speech recognition system did not get that
much more accurate, or your online advertising
system didn’t get that much more accurate that’s
showing the most relevant ads, even as you show the more data. AI has really taken
off recently due to the rise of neural networks
and deep learning. I’ll define these terms more
precise in later video, so don’t worry too much
about what it means for now. But with modern AI, with neural networks
and deep learning, what we saw was that, if you train
a small neural network, then the performance
looks like this, where as you feed them more data, performance keeps getting
better for much longer. If you train a even slightly
larger neural network, say medium-sized neural net, then the performance
may look like that. If you train a very
large neural network, then the performance just keeps on getting
better and better. For applications like
speech recognition, online advertising,
building self-driving car, where having a high-performance, highly accurate, say speech recognition system
is important, enable these AI systems
get much better, and make speech
recognition products much more acceptable to users, much more valuable to
companies and to users. Now, a few couple of
implications of this figure. If you want the best possible
levels of performance, your performance to be up here, to hit this level of performance, then you need two things: One is, it really helps to
have a lot of data.
Source
What is Machine Learning? | Andrew ng| tejascoursera| deepalearning.ai
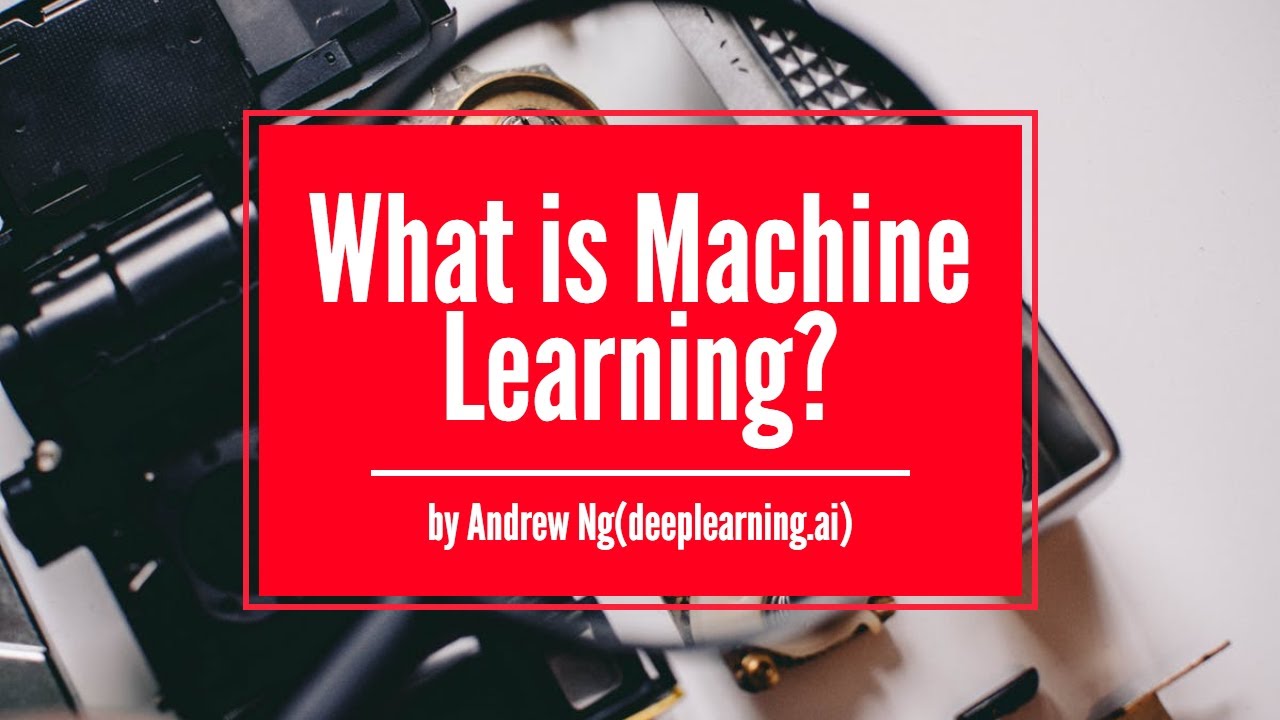